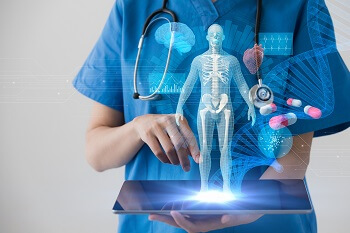
8/24/2020
In its effort to characterize the diverse methods, applications, and perspectives of explainable Artificial Intelligence (AI), the National Institute of Standards and Technology (NIST) is seeking comments on its white paper, "Four Principles of Explainable Artificial Intelligence." The American National Standards Institute (ANSI) encourages its relevant stakeholders to submit feedback via NIST's call for comments page by the October 15 deadline.
NIST's paper presents four "principles" that capture the fundamental properties of explainable AI systems. Each of these principles, as NIST explains, are heavily influenced by an AI system's interaction with the human receiving the information. "The requirements of the given application, the task, and the consumer of the explanation will influence the type of explanation deemed appropriate," according to NIST. Furthermore, NIST explains that its four principles are intended to capture a broad set of motivations, applications, and perspectives.
The Four Principles of Explainable AI
-Explanation: Systems deliver accompanying evidence or reason(s) for all outputs.
-Meaningful: Systems provide explanations that are understandable to individual users.
-Explanation Accuracy: The explanation correctly reflects the system's process for generating the output.
-Knowledge Limits: The system only operates under conditions for which it was designed or when the system reaches a sufficient confidence in its output.
Read more about the four principles in more defined and contextualized detail in NIST's white paper.
What is Explainable AI?
As NIST explains, AI must be "explainable" to society to enable understanding, trust, and adoption of new AI technologies, the decisions produced, or guidance provided by AI systems.
Read about ANSI member efforts in AI, and related news:
Standards Support Advancements in Artificial Intelligence for Healthcare
USNC Current Newsletter Focuses on Artificial Intelligence and Automated Systems